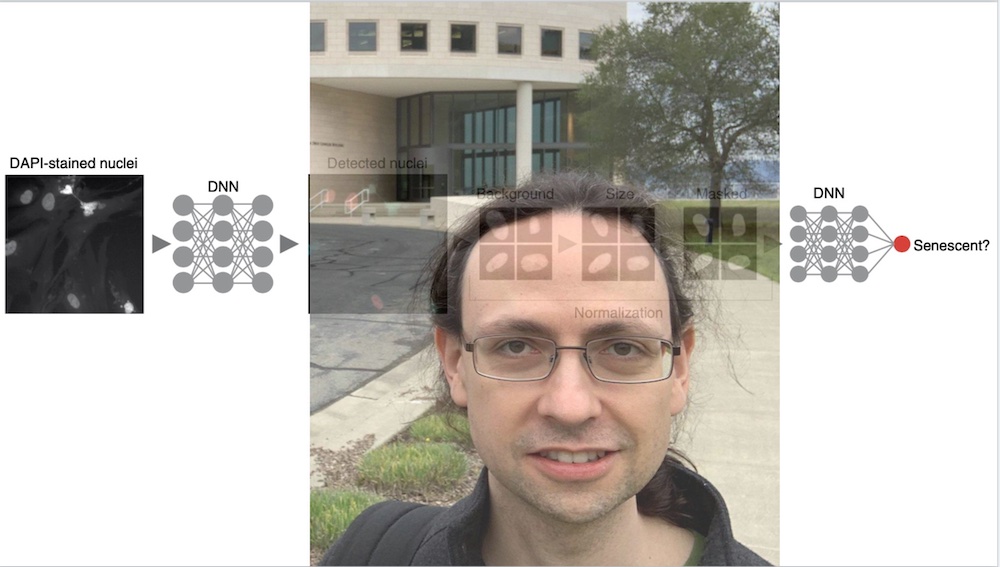
Deep Learning Predictor Can Tell The Age of Your Cells
When you age, your cells also age. It has long been a challenge for scientists to identify the cells that are aging, or reaching cellular senescence, as it is called. To understand aging and age-related diseases, there’s a need to understand how to detect cellular senescence.
This is what Indra Heckenbach is studying. In a recent paper (the first paper in his PhD) in Nature Aging he shows how neural networks can be used to predict senescence with up to 95% accuracy.
“The cells that I have been looking at are from Patobank at Rigshospitalet. 169 skin biopsies. I studied the images of the tissue, used methods developed in the paper, and then compared those results with the health outcomes of the 169 individuals,” Indra Heckenbach explains from his home in California where he’s been living for the past two year, and where he – besides working on his PhD – is also working with the Buck Institute, collaborating on several research projects.
As part of a Novo Nordisk Foundation Challenge project, Heckenbach began by investigating tissues in dermal biopsy images from Denmark’s Pathology Specimen Biobank (Patobank). After exploring age-related changes, he wondered if senescence could be detected. He turned to cells in culture, where you can induce and detect senescence more directly. He found that neural networks could be readily trained on raw images but struggled to apply them to tissues. Trying to map the predictor models to tissue, he found a set of simple normalizations, after which the predictor showed significant differences by age. He later confirmed it was detecting senescence through several experiments with collaborators. Evaluating other species and cell types, he found it detected senescence in all cell types tested for human and mouse, suggesting that the deep learning predictor was identifying a common signature of senescence.
According to Ming Xu, an expert in Nature Aging, the study makes an important step forward and the work could serve as a proof-of-concept evidence that deep neural networks can be developed to detect senescent cells.
“With more senescent cells, you have lower risk for cancer. But the flip side is that these cells lead to damage in tissues and are associated with many diseases of aging like osteoporosis, hypertension and other diseases of aging,” according to Indra Heckenbach.

His work takes a lot of in-depth analysis and a lot of iteration. You try one thing and it doesn’t work, and then you try another approach.
“We had a lot of positive results early on, and that was encouraging. We were able to automatically identify senescence in vitro, that is in the lab, and no-one had done that before,” says Heckenbach answering what keeps him going.
According to Heckenbach and his advisor, aging and DNA scientist Morten Scheibye-Knudsen, also from the Center for Healthy Aging at Copenhagen University, there are several implications of the work.
The senescence predictor and related methods could potentially assist in several directions, including drug discovery, assessment of treatments, and better characterization of senescence in tissues related to aging and disease. It might even lead to a deeper understanding of the senescent phenotype given the apparently conserved morphological changes being detected across tissues and species, they say.